- The Augmented Advantage
- Posts
- Winning RFPs with AI
Winning RFPs with AI
How to find profitable AI use cases that enhance your RFP process
Hi there,
I recently had the amazing opportunity to speak at the Association of Proposal Management Professionals (APMP), which has more than 10,000 members worldwide.
The big question was - how does (generative) AI affect our work? Does it at all?
Today I'd like to share with you the process of finding a good use case for it.
Let's get started!
Do you enjoy reading this newsletter?
If so, please leave a quick testimonial - it would mean a lot to me!
Common Misconceptions About AI
When exploring how AI can optimize the RFP response process, it's important to clear up some common misconceptions, such as:
āAI will replace human jobsā - In reality, AI augments human capabilities, at least in the short term.
āAI is too expensiveā - If you start the right way, the long-term benefits can tremendously outweigh the costs
āAI is too complexā - Modern AI solutions are becoming more commoditized by the day and designed for ease of use.
āOne AI solution fits allā - In fact, different tasks require different AI approaches
Dispelling these myths is key for effective AI adoption.
Finding AI Opportunities in the RFP Response Process
One of the best ways of finding profitable AI opportunities is to look at it from a business process angle.
The overall idea is simple.
List out one or many business processes, identify pain points and see how AI capabilities can help.
For Proposal Management Professionals there are tons of different business processes they deal with - responding to requests for proposals (RFPs), doing market analysis, client engagement, etc.
For this newsletter, letās just focus on one aspect: Responding to RFPs
Step 1: Mapping the business process
If we zoom in on the āResponding to RFPā process, there are a couple of sub-steps involved.
For example, a typical RFP response process could involves key stages like this:
Analyzing the RFP and assembling a team
Developing a proposal outline and plan
Drafting, customizing and reviewing content
Finalizing formatting, gaining approvals, and submitting on time
Following up with the client afterwards, and debrief internally
When mapping the process steps, try to find a good balance between being specific enough to be actionable, but not getting lost in the details.
Step 2: Find Pain Points
The next step is to identify pain points that appear at each step.
This can be things that slow down the process, cost a lot of money or just cause a poor customer experience.
In our RFP example, the pain points could be:
Difficulty quickly digesting complex RFPs
Struggling to write persuasive, customized content
Ensuring proposal compliance and consistency
Formatting issues and delays in internal approvals
Collecting constructive client feedback after submission
These challenges can be roadblocks to efficiently developing winning proposals.
(Of course, you should customize these pain points to you.)
So how could we use AI to overcome the pain points above?
Step 3: Mapping AI Capabilities to Optimize the Process
Letās see how we can map high-level AI capabilities to potentially help us solve our problems.
A simple table will do the job for us to get started:
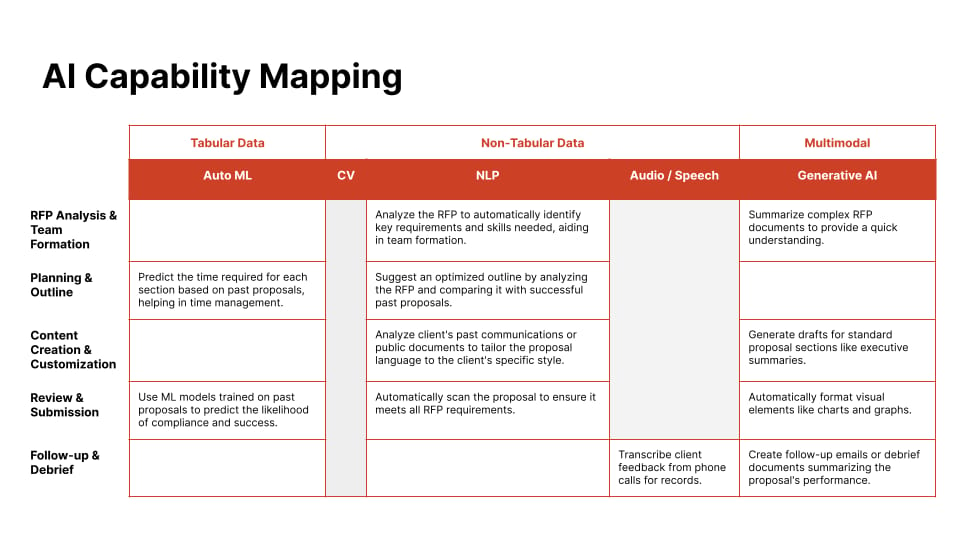
Of course, if you would do this in a real scenario, you would go even deeper here. For example, if we did this as part of an AI Design Sprintā¢, we would use the AI cards instead of only the 5 main AI categories:
In our example, a quick analysis would show us that mainly Generative AI as well as NLP and perhaps even some Auto ML technology could help us solve some critical pain points.
Step 4: Identifying and Prioritizing AI Use Cases
With our list of potential use cases we can now go ahead and determine where to focus our AI efforts.
To do this, weāll:
Write out potential use cases and their impact vs. feasibility
Prioritize those with high business value and implementation ease
Prototype top choices quickly to demonstrate benefits
The strategy: Starting with quick wins builds momentum.
For every potential use case we would come up with a quick use case fact sheet like this:

Based on the fact sheets, every use case would have an expected feasibility and business value score - rough cuts are enough at this stage.
Our goal would be to classify use cases by low-medium-high feasibility and business value first.
This results in a 3Ć3 use case matrix like this one:

With that matrix, we usually have a few potential candidates to pursue that have at least medium impact and medium feasibility.
So which one to build?
Step 5: Prototyping for Quick Wins
For our first prototype, weāre looking for so-called Atomic AI use cases. These are use cases that are easy to build because they require a low amount of integration and we can leverage off-the-shelf tools.
In our example here, good use cases to prototype first include:
Summarizing RFP documents with generative AI
Checking proposals for compliance with NLP
Transcribing client feedback using speech recognition
These can be piloted rapidly with minimal integration needs.
If we were a group of business decision makers, we could vote which one to pursue.
Iād probably go with the Summarizing RFP documents with Generative AI one!
Instead of having 30+ pages of documents to read through, why not simply build a short summary or even small chatbot that can answer the most common questions about that RFP?
Before:

After:

Hereās a video of how I created the chatbot in less than 5 minutes:
This chatbot would help the team save a lot of time answering common questions about the given RFP project ā and itās multilingual out of the box!
Step 6: Iterate for the win!
We've quickly built a first chatbot, but this is by no means the final version.
If the prototype piques the curiosity and interest of the intended users, it's probably worth developing further.
Our goal is to improve it by adding features like reliable sources, data security, and integration with internal data sources. Hereās an example video how this could look like.
We would release features gradually to ensure that the chatbot is valuable.
I can't stress this enough:
It's important to have an iterative mindset before you start any AI projects.
AI and data projects inherently carry a high degree of uncertainty across many dimensions, which can only be addressed with an agile approach.
Key Takeaways
If you thoughtfully apply the right AI technologies at different stages of the RFP process, you can gain real advantage as a proposal management professional.
By taking an incremental approach that focuses on impactful, feasible use cases and rapid prototyping, you can continually optimize a complex process and deliver better proposals.
I hope you found this newsletter useful. If so - please leave a feedback! ā¤ļø
For any other questions, feel free to reach out!
I'm always happy to discuss how AI can augment and improve your business.
See you next week!
Tobias
Want to grow your business with AI? Here are 3 ways I can help:
Book a meeting: Let's find out how I can help you over a coffee chat (use code FREEFLOW to book the call for free).
Read my book: Improve your AI/ML skills and apply them to real-world use cases with AI-Powered Business Intelligence (O'Reilly).
Follow me: I regularly share free content on LinkedIn and X.
Reply