- The Augmented Advantage
- Posts
- 4 Proven AI Adoption Approaches for "Normal" Businesses
4 Proven AI Adoption Approaches for "Normal" Businesses
How to Find Your Own Path Without Copying Silicon Valley
When someone asks me, "What's the best way to adopt AI?" I often find myself saying, "It depends".
But what does it depend on? We’ll get to that in a bit.
One thing I can tell you for sure: copying what the so-called "AI experts" in Silicon Valley are doing isn’t the answer.
It might sound counterintuitive, but as you'll see in this article, following in the footsteps of big software companies is rarely the best approach to AI. Instead, I’ll share with you four strategies that actually work for "normal" businesses, so you’ll have a clearer idea of what might work for you.
Let’s jump in!
Check out today’s sponsor:
The fastest way to build AI apps
Writer Framework: build Python apps with drag-and-drop UI
API and SDKs to integrate into your codebase
Intuitive no-code tools for business users
Why Following Big Tech's Footsteps is a Mistake (A.K.A The Copycat Trap)
There's a strange phenomenon in the business world where companies - especially those with low tech and high legacy - mimic the practices of Silicon Valley software giants, hoping to replicate their success. This isn't limited to AI. Take OKRs (Objectives and Key Results) as an example. Google made them famous, and suddenly, there was a phase where everyone wanted to do it. After all, if Google does it, it must be good, right? Turns out, maybe not.
(Same with agile, but don't get me started on that).
Now, the same misguided approach is happening with AI. Companies around the world think that approaching AI the way big software companies are doing it – which essentially boils down to throwing an absurd amount of resources at a problem and see what sticks – will work well for them, too.
What they don't realize is that these Silicon Valley tech giants are playing a different game. There's probably a 0.01% chance that you can keep up with the talent, money, and AI experience these companies put into their AI roadmap.
It's crucial to find an AI strategy that aligns with your specific context. But the good news is, you don't have to start from scratch. In fact, across all the companies I've worked with, I've seen four key patterns emerge in how companies are successfully approaching AI adoption.
So let's learn more about them, and how popular winners in each category look like.
Approach 1: "Divide and Conquer"

How it works: The key situation here is that you’re generally AI-optimistic and in an industry that is highly impacted by AI. Your focus is on spreading AI adoption quickly and horizontally across the whole organization. To achieve this, you act like the Romans: empower different parts of your empire to operate independently while ensuring they all obey a larger common goal. With AI, this means launching multiple small projects that collectively build momentum on a strategically aligned roadmap, typically orchestrated by an AICoE or similar. In a "Divide and Conquer" approach, it’s not uncommon to find a backlog of 200+ use cases. While each of them has a relatively low impact individually, together they build towards a larger strategic vision. This key distinction separates this approach from letting everyone in your organization pursue their own AI projects without oversight, which will result in a death by 1,000 prototypes.
Benefits:
Drive efficiency and innovation across the organization while upskilling employees on the new technology.
High employee engagement and supporting a culture of innovation.
Easier to scale and adapt without the risk of a single large-scale failure.
Limitations:
Harder to quantify overall value.
Ensuring alignment across departments can be complex.
Use cases will most likely need to be funded from current budgets because there is no overarching business case.
Case Study: Moderna

Biotech innovator Moderna onboarded over 80% of their employees on using AI tools that augment employees' roles across various functions like legal, R&D, manufacturing, and commercial to drive employee productivity and increase the competitiveness of the overall business.
Approach 2: "AI Killer Use Case"

How it works: Unlike driving AI enablement across your organization for various internal use cases, the "AI Killer Use Case" approach rallies your team around a single, transformative AI solution that, if realized, could significantly reshape your business. This use case becomes a cornerstone of your overall strategy, drawing continuous C-level attention. Killer use cases often require deep vertical integration and combined efforts from multiple departments, along with substantial R&D investments. To make this big effort possible, you’ll likely need to adjust your organizational structure to ensure alignment, ownership, sponsorship, and effective risk management.
Benefits:
Can redefine entire industries and establish new leadership.
If successful, creates a massive competitive moat.
Potential to transform operations and value proposition and unlock completely new business models.
Limitations:
High Risk
Requires substantial investment in capital, talent, and time
Regulatory approval might apply
Case Study: Tesla

Tesla’s AI killer use case is autonomous driving. If they can crack it, they’ll transform into a global shared mobility provider virtually overnight, unlocking entirely new revenue streams beyond simply selling cars. Autonomous driving also allows them to charge a premium for their vehicles, positioning them as not just a car manufacturer, but a leader in cutting-edge technology. To make this vision a reality, Tesla maintains strong vertical AI ownership across the entire value chain—from custom AI chips and massive data collection efforts to top-tier talent and advanced algorithms. However, this ambitious focus comes with significant risk: if they fail to deliver on this use case, the entire business model is at stake.
Approach 3: "Product-Led AI"

How it works: This approach looks at AI mainly from a product perspective. Instead of overhauling your business model, it focuses on enhancing existing products with AI to boost functionality and user experience, helping you stay ahead in competitive markets. Often, there’s no need to highlight the AI aspect— your products take center stage, with AI seamlessly integrated to enhance their value and performance.
Benefits:
Enhance product value through new features and/or improved user experience.
Drive customer satisfaction and loyalty.
Differentiate products in crowded markets.
Limitations:
AI stays an enhancement, not a core differentiator and competitors might be able to quickly replicate AI-driven features.
Impact is typically confined to product-level improvement with less organizational learning.
Continuous innovation is needed to stay competitive.
Case Study: Apple
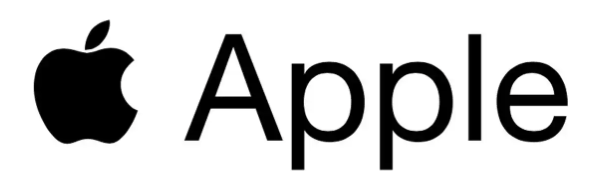
Apple’s approach to AI is a textbook case of product-first integration. They hardly mentioned AI until WWDC 2024, yet they’ve consistently enhanced the user experience without overhauling their business model. Features like FaceID offer seamless security, and machine learning models have significantly improved photo quality—helping Apple maintain its market leadership. Augmented AI features like the following show their commitment to this subtle, product-centric approach:
Approach 4: "Selective AI"

How it works: This approach works well for industries that aren't significantly impacted by AI and can afford to take a wait-and-see approach. You hold off on AI adoption until there's a use case so compelling that it can't be ignored. This means being extremely efficiency-oriented and carefully selecting only proven AI applications that deliver immediate, tangible benefits. By watching the market to see what works for others, you make quick, calculated moves where others have successfully tested the waters. Typical use cases can be found in general functions such as marketing, sales, customer support, and others that I've described here.
Benefits:
Lower-risk investments
Quick return on investment
Reap AI benefits without trial and error
Limitations:
Less potential for true innovation, always playing catch-up
May miss out on transformative opportunities.
Risk of becoming too comfortable with existing systems.
May fall behind more aggressive competitors.
Case Study: The Home Depot

The Home Depot - exemplary for many other retailers - is a good example of selective AI adoption. (At least given what they share publicly.) By leveraging proven technologies like supervised machine learning and computer vision, they help employees manage inventory better, which improves both in-store and online shop performance, and enhance their online search experience so customers can find products more easily.
Final Thoughts
Can you mix and match these approaches? Of course, but be sure not to fritter away in details. Choose your primary focus and let that be your north star. Feel free to add other elements here and there, but always keep your main objective clear.
As you consider your next steps, ask yourself: Which of these four approaches resonates most with you? Why?
Making the right turn at this critical intersection is crucial to your future AI and business success.
Reach out if you need help.
Otherwise, I'll see you next Friday!
Tobias
PS: Do you enjoy reading my newsletter? I'd love to hear about it!
Reply